Unlocking Insights into Employee Turnover: A Data-Driven Approach at Company X
Sep 24, 2024
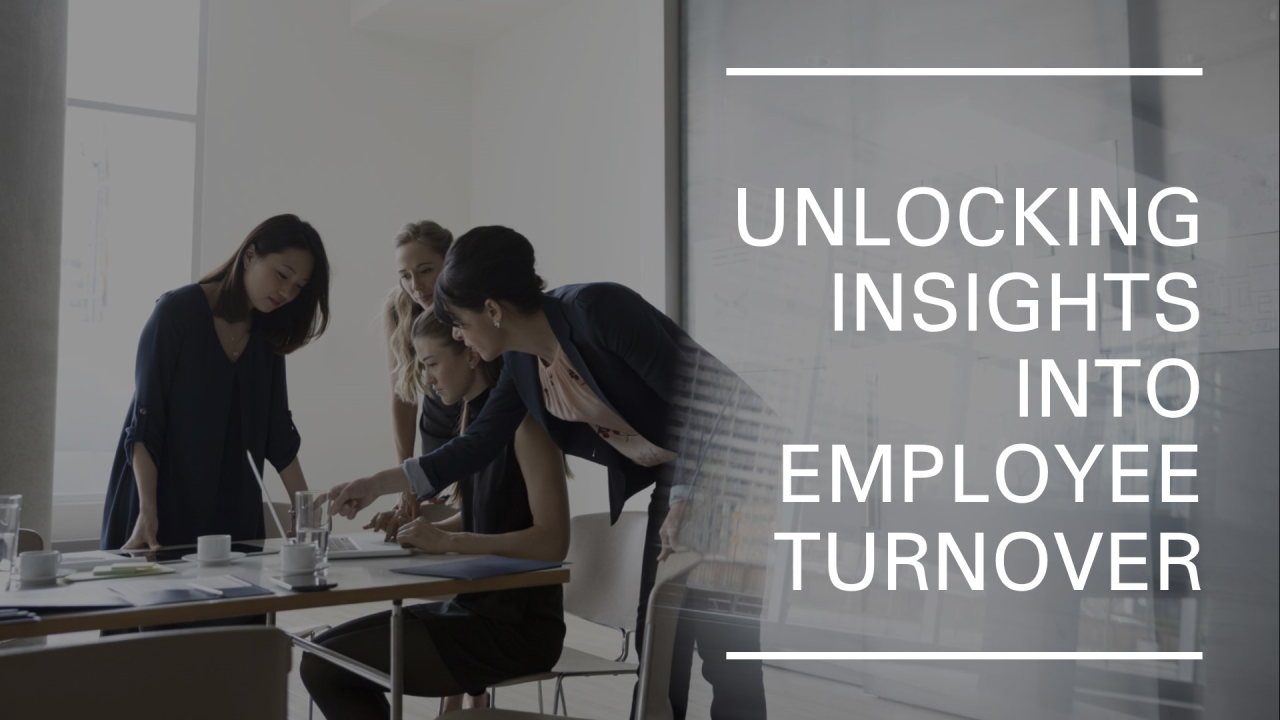
In today's dynamic work environment, retaining top talent is a critical challenge for organizations. The recent study "Predicting and Mitigating Employee Turnover at Company X" provides valuable insights into how data-driven strategies can address this challenge. As professionals increasingly recognize the importance of employee retention, this research offers a blueprint for leveraging machine learning to enhance workforce stability.
The Study's Scope and Findings
The study focuses on identifying the key factors that contribute to employee turnover at Company X, an innovator in app development. By analyzing a range of employee data—such as satisfaction levels, promotion history, work-life balance, and workload—the research aims to predict turnover and propose effective retention strategies.
Key Findings:
- Critical Factors: The study highlights that job satisfaction, promotion frequency, and workload balance are significant predictors of turnover. Employees with low satisfaction, limited career advancement opportunities, or imbalanced workloads are more likely to leave.
- Model Efficacy: Among the machine learning models tested, the random forest classifier stood out with the highest accuracy and AUC, proving to be the most effective in predicting turnover at Company X.
- Retention Strategies: Based on the predictive model's outcomes, the study categorizes employees into different risk zones. It recommends tailored strategies for each zone, ranging from enhanced engagement programs to targeted interventions for high-risk individuals.
Implications for HR and Management
This research underscores the value of using advanced analytics in HR practices. By adopting data-driven approaches, companies can not only predict potential turnover but also implement proactive measures to retain valuable employees. The study suggests that promoting career development, recognizing employee achievements, and maintaining a balanced workload are crucial steps in this direction.
For HR professionals and management teams, these findings offer a practical framework for developing and refining retention strategies. The use of machine learning models, like the random forest classifier, provides a robust tool for anticipating employee needs and addressing potential issues before they lead to turnover.
A Broader Perspective
While the study focuses on Company X, its implications are far-reaching. Organizations across industries can benefit from integrating similar predictive models into their HR analytics toolkit. As we move toward a more data-centric approach in all business functions, the ability to foresee and mitigate employee turnover will become increasingly vital.
In conclusion, the study does not only contribute to the academic discourse on employee retention but also provides actionable insights for practitioners. It highlights the power of combining human insight with technological tools to create a more stable and satisfied workforce.
As we continue to explore innovative ways to retain top talent, this study serves as a timely reminder of the importance of data in decision-making. Let's continue the conversation on how we can leverage these insights to build better workplaces and stronger teams.
Share this Article